Marcin Feder, Leader and manager of Data Analysis and Molecular Modeling Laboratory, Adamed Pharma
Artificial Intelligence (AI) is one of the buzz terms that recently spread on headlines across both popular and speciality media. We’ve learned that AI can drive cars better than an average human, beat the best of us in games of chess and Go, compose music and even be appointed to the board of directors in a venture capital firm.
Yet, in a more subtle way, AI algorithms have already started to interfere with our everyday life. Virtual assistants suggest possible answers for messages we get in various communicators, fix our spelling and grammar, recognize our speech, recommend the next movie we would like to watch or the products we would like to buy. All of these are far from trivial tasks, even for humans. Thus, it seems reasonable to expect that these AI algorithms will also conquer other fields that require both broad expertise and the ability to make rational decisions based on a wide range of data. Drug discovery seems to be the perfect application for these methods, so can they already support or maybe even replace “organic” drug hunters?
What we usually mean when we talk about AI
When someone uses the term “Artificial Intelligence” we usually think of “machines” that can perform human-like tasks. They can “understand” our questions and provide reasonable answers such as “where is the nearest barber shop? ”, identify pictures containing “cute kitties” or control virtual characters in computer games. These algorithms are already so sophisticated that may leave us with the disturbing sensation that we have just interacted with some kind of “intelligence”.
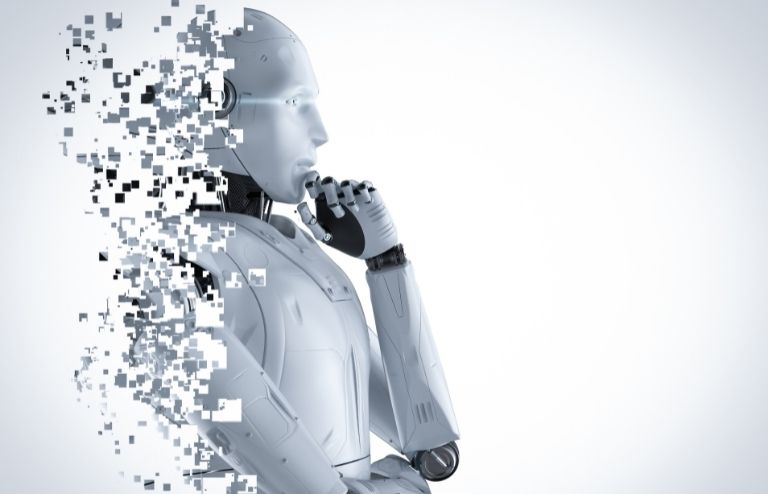
This perception isn’t completely wrong but it may be a little bit misleading, as the most distinguishing feature of AI algorithms is hidden behind the curtain. In the process of their creation, they are given the ability to learn – specifically from examples. Most of the algorithms we use in our “traditional” software were encoded based on the knowledge of their designers. For example, to create an email validator, we can define a set of rules that will identify whether a given string of letters is a valid email address or not. For this purpose, we could come up with a simple formula that will check whether a string has exactly one @ character, at least one dot, a valid domain name etc.
The AI way of solving the same problem will be completely different. Instead of applying our own knowledge on how a proper email address should look like, we would rather build a dataset of real email addresses and let our program infer the rules itself. In order to make the learning process more effective, we would also probably provide our AI with a negative training set containing strings that are everything but valid email addresses.
Self-learning algorithms in computer science have been continuously developed for over fifty years and they are called Machine Learning methods (ML). For a long time, they were mainly utilized in narrow, specialized applications and recognised only by scientists. With the increased digitization of our world, this situation has started to change. A combination of several significant technological improvements, both in the methods themselves and more general IT technologies, and the growing availability of large data sets related to real life problems has resulted in rapid growth for Artificial Intelligence. The sophisticated ML algorithms can infer patterns in raw data and encode them in “models” that can later be used to solve classification or prediction problems.
Machine Learning in drug discovery
Machine Learning has a long history of application in chemoinformatics. At the beginning, they were mainly employed to predict various properties of potential drug candidates as a part of a quantitative structure-activity/property relationship (QSAR,QSPR) approach. In QSAR/QSPR modelling, scientists try to correlate selected, experimentally measured properties like drug solubility, absorption, toxicity or biological activity with the various “features” of the chemical structure called descriptors. The descriptors are mathematical values that encode miscellaneous features derived from chemical formulas or structures, like the number of atoms, charge, the presence of specific groups or atom connectivity.
In practice, preparing such a model requires several steps. Let’s assume we would like to build a solubility predictor. Then, having a data set of compounds with experimentally measured solubility, we need to:
- Compute descriptors for all compounds in a dataset and split the data into separate training and test sets (data processing phase)
- Identify which subset of descriptors has the highest influence on measured solubility (feature extraction phase)
- Optimize a mathematical function that takes a set of descriptors for input and returns the predicted solubility as the output. This process aims to achieve the highest possible correlation of predicted and measured values for the training set (model development or training phase)
- Validate whether the “model” obtained in the previous step can predict the solubility of compounds from the test set that were not included in the training process (model validation phase)
Such a model can then be later used to predict the solubility of newly designed compounds and prioritize the synthesis of the most promising ones. In this way, scientists in pharma companies and academia routinely build numerous QSAR/QSPR models that are used to guide the optimization of drug candidate selection.
From shallow to deep learning
The early QSAR models were primarily based on linear regression methods. However, a high dimensionality of data and a high complexity of modelled relationships proved hard to tackle with these simple regression methods. Thus, the cheminformatics field has quickly adapted dimensional reduction techniques like principal component analysis (PCA), as well as a full range of non-linear classification ML methods, including Support Vector Machines (SVM), decision trees, Bayesian classifiers and various artificial neural networks (ANN). All of these traditional “shallow” methods are still widely used in modern computer-aided drug discovery and have been successfully applied in numerous research programs.
In the meantime, as a direct extension of ANN, several deep learning techniques like deep neural network (DNN) have been introduced to process high dimensional data, as well as unstructured data for machine vision and natural language processing. The practical framework of DNN was created by Hinton, LeCun and other scientists in 2006, opening the revolutionary waves of Deep Learning and a new AI. They proposed a novel architecture for multilayer ANNs that introduced two major new developments: feature learning and several levels of data abstraction.
Through feature learning, DNN methods could automatically extract features from input data with raw format, then transform and distribute them into more abstract levels. This practically allows such processes to skip the separate data abstraction phase and significantly improve predictive efficacy for very complex properties, but only on the premise that the training set contains a tremendous amount of data. With very limited data, the DNN cannot achieve an unbiased estimate of the generalization, so they may not be as practical as some traditional shallow ML methods. Also, with a rapid increase in time complexity because of the complication of the network architecture, stronger hardware facilities and advanced programming skills are required to grant the feasibility and effectiveness of Deep Learning.
Data, data, data
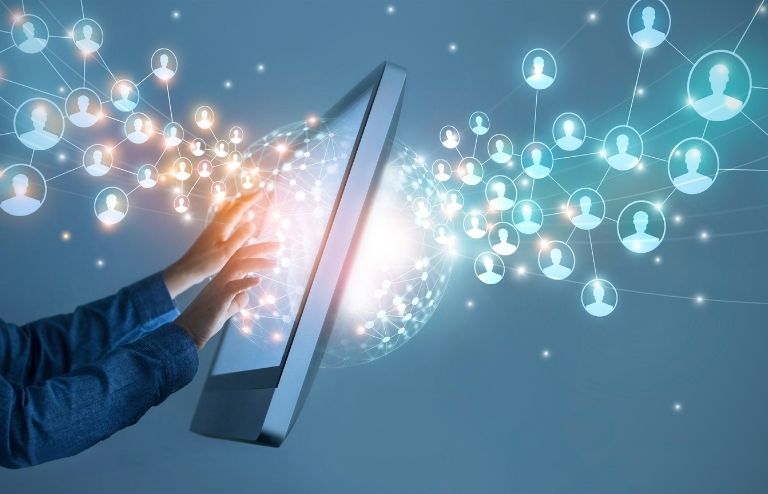
The increasing popularity of AI technology and the public availability of leading edge Deep Learning frameworks, such as Facebook’s Torch or Google’s TensorFlow, have enabled even higher adoption of ML methods in drug discovery. Notably, it has breathed a new life into the areas going beyond QSAR, like predicting drug-target interactions, the de novo design of bioactive compounds, proposing novel routes of chemical synthesis or suggesting drug targets and biomarkers based on genetic associations. Looking at the dynamics of these developments, one would expect that AI technologies will cover all the aspects of drug discovery. That, in the near future, drugs will be developed through an automated platform that integrates theoretical computation results, genomics, chemistry and biomedical data. However, in reality, we are still far from this point, as a critical barrier for further improvement can be found in the availability of high-quality, well curated data in large quantities necessary for training and developing accurate ML models.
The complexity of pharmaceutically relevant systems sets extremely high requirements for the amounts and accuracy of the training data sets. This is where governments, academia and a pre-competitive consortia of pharmaceutical companies and academic institutions that use appropriate data standards, and have the necessary operational and open data frameworks, could be part of the solution to meet these data demands. Without such initiatives, we won’t be able to fully utilize the potential of the AI revolution in drug discovery.
Adamed Pharma is a partner of MIT EF CEE Autumn 2021 acceleration program. Want to know more about them? Check out what are they looking for in the startup world.
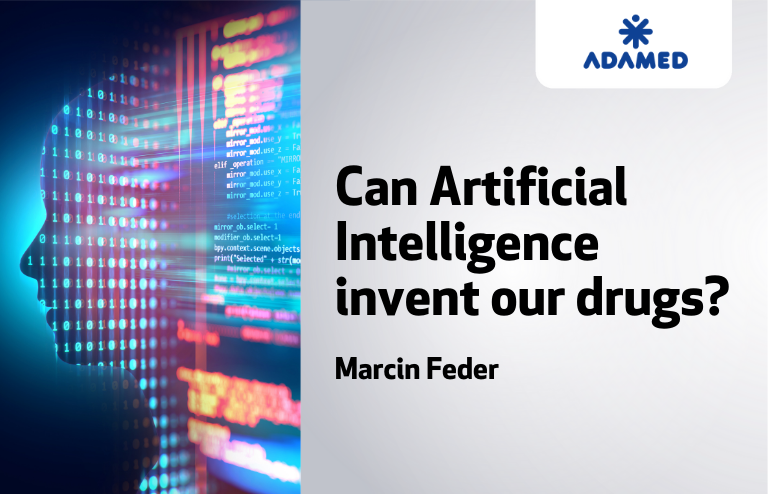